Keynote Speakers
Preliminary Schedule
Name | Schedule | Room | Slides | Video |
---|---|---|---|---|
Gerhard Widmer | Monday 18.00 | Festsaal 1 Library and Learning Center (LC) Vienna University of Economics (WU) | Video | |
Tim Miller | Tuesday 09.45 | Strauss 2-3 | Video | |
Pete Wurman | Tuesday 14.00 | Strauss 2-3 | Video | |
Jérôme Lang | Wednesday 9.00 | Strauss 2-3 | Download | Video |
Sumit Gulwani | Wednesday 14.00 | Strauss 2-3 | Video | |
Judea Pearl | Thursday 9.00 | Strauss 2-3 | Video | |
Mihaela van der Schaar | Thursday 14.00 | Strauss 2-3 | Video | |
Ana Paiva | Friday 9.00 | Strauss 2-3 | Video |
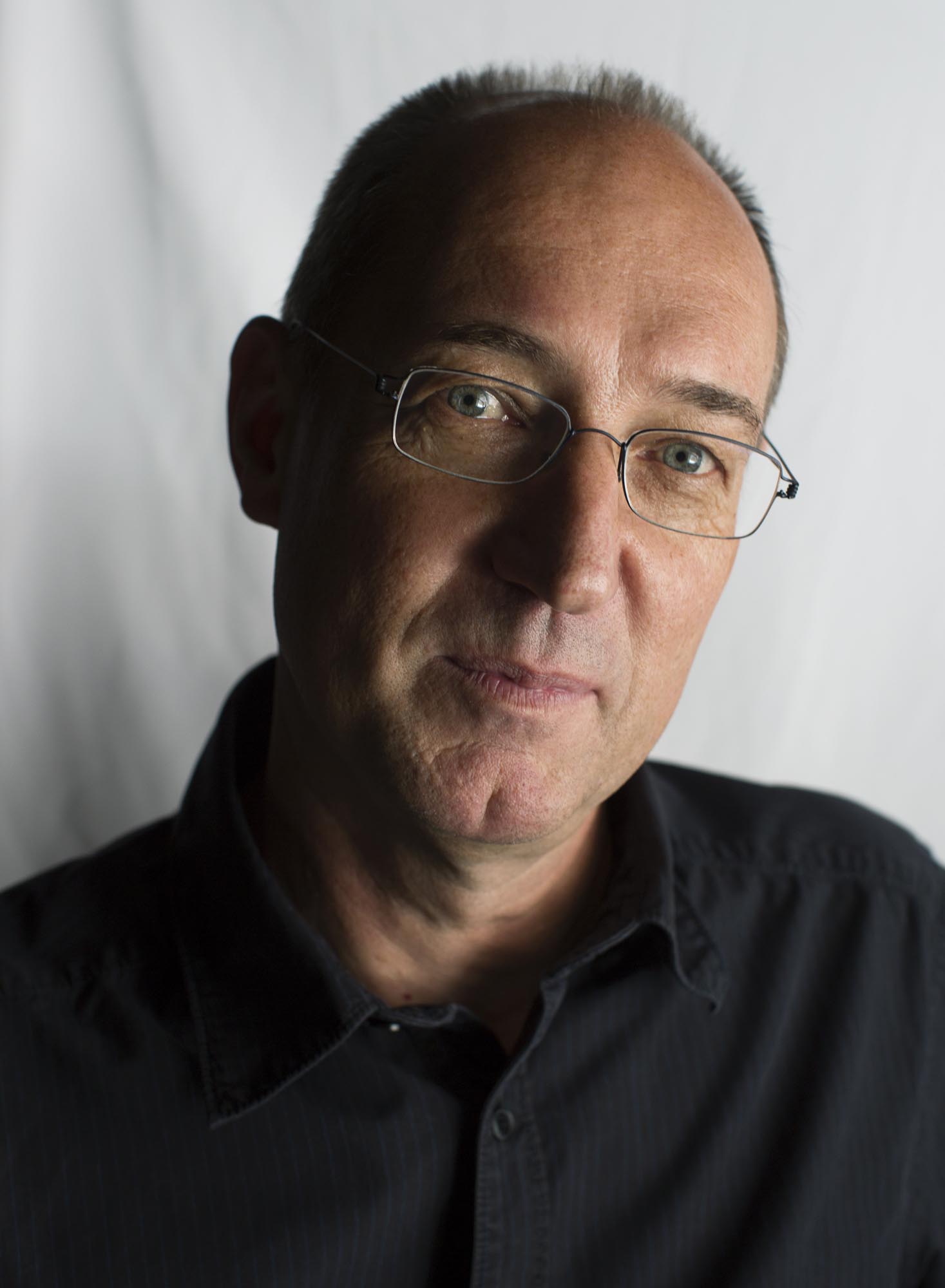
Gerhard Widmer
Title: AI & Music: On the Role of AI in Studying a Human Art Form
Abstract: The field of AI & Music has come a long way, from early attempts at algorithmic composition to a wide variety of intelligent sound and music technologies that are shaping today’s digital music world. As in many other application domains, many of the recent successes are based on exploiting and adapting the latest advances in statistical machine learning, transferring them from fields like computer vision and language processing.
But music is much more than just an acoustic signal with hidden patterns, or a sequence of events with statistical properties. Music is a deeply human art, a unique means of human self-expression and emotional communication, and we still lack a full understanding of how music “works”, as a communication language between composers, performers, and listeners.
I will try to demonstrate that AI and machine learning may have something to contribute here, by providing means of studying some of these questions via computational modeling. We will focus on the complex phenomenon of
expressivity in music performance (of classical music): how skilled performers make music “come alive” and communicate subtle aspects like moods, ideas, emotions through their playing. I will take the audience through a recent big research project that aimed at modeling certain aspects of this with machine learning. We will see what it takes to model and explore such an elusive concept in a data-driven way, talk about the difficulty of obtaining reliable data, but also about the difficulty of evaluating the results, and how we may nevertheless obtain some interesting and possibly useful results. We will see (and hear) computer models of expressive playing — including, if circumstances permit, a live demonstration of human-machine piano co-performance –, and we will even learn about an expressive performance system that allegedly passed a musical “Turing Test”. However, we will also find (or I will contend) that the very idea of a “Turing Test” in this context (and perhaps generally in the arts) may be fundamentally problematic. At a more general level, I will argue that we should carefully consider the proper role of AI in music, and what claims we can justifiably make about AI & music systems, if we take music seriously as an expressive (human) art form.
Bio: Gerhard Widmer should have become a pianist, but at age 15 he decided that Beethoven was boring and instead studied computer science in Vienna, Austria and Madison, Wisconsin (USA). He is a full professor of computer science at Johannes Kepler University Linz, Austria, where he founded the Institute of Computational Perception, as well as deputy director of the LIT AI Lab and a member of the ELLIS Unit Linz. His research interests are in AI, machine learning, acoustic perception, and computational models of music. He is considered one of the pioneers of the field of AI & Music and was centrally involved in the organisation of some of the earliest international AI & Music workshops (e.g., at ECAI 1992 and IJCAI 1995). He is particularly well known for pioneering work on computational modelling and analysis of expressive music performance.
Widmer has served on the editorial boards of journals such as Machine Learning, Journal of AI Research (JAIR), Journal of New Music Research, and on the advisory board of AAAI Press, and has given keynote talks at many leading conferences in the fields of AI (IJCAI, ECAI), machine learning (ICML, ECML, ALT), and cognitive/empirical musicology (ICMPC, ESCOM). Likewise, his work has been published in the top journals of these fields. At the same time, his teams have also developed commercial applications in the digital music and media worlds (e.g., the MOTS technology integrated in some of Bang & Olufsen’s digital media players, and music detection and audio segmentation algorithms that are being used in a variety of media monitoring contexts, worldwide).
Widmer is a member of the Executive Board of the International Research Center for Cultural Studies (IFK), Vienna, a EurAI and an ELLIS Fellow, and has received many prestigious research grants and awards (including Austria’s highest scientific award, the Wittgenstein Prize; two ERC Advanced Grants of the European Research Council; and the “Scientific Breakthrough of the Year 2021” award of the Falling Walls Foundation). In 2021, he was the first person, in the 175 history of the academy, to be simultaneously elected into both classes (Mathematics & Natural Sciences; Humanities & Social Sciences) of the Austrian Academy of Sciences. His attitude towards Beethoven has also changed substantially in the meantime.
Note that this talk takes place at Festsaal 1, Library and Learning Center (LC), Vienna University of Economics (WU). There is limited seating capacity, but streaming to the aula of building is provided. Subsequent to the talk, the opening reception will take place in the same building.
Tim Miller
University of Melbourne, Australia
Title: Are the inmates still running the asylum? Explainable AI is dead, long live Explainable AI!
Abstract: In this talk, I will discuss why I believe many of the assumptions underlying explainable AI are misguided, and how we can address this issue. In the past, I have argued that in the explainable AI community, maybe ‘the inmates are running the asylum’, re-framing Alan Cooper. By this, I mean that experts in artificial intelligence are not well placed to design explainability tools and techniques that are intended for non-expert users, and we should turn to the social sciences and human-computer interaction to mitigate this. I will review discuss theories from philosophy, cognitive science, and social & cognitive psychology that have been influential in explainable AI in the last few years, improving explainable techniques. I’ll discuss that, despite this progress, I believe the inmates have been running the asylum all along without us knowing, but that by questioning our assumptions, we can change direction to see improved outcomes.
Bio: Tim is a professor of computer science in the School of Computing and Information Systems at The University of Melbourne, and Co-Director for the Centre of AI and Digital Ethics. His primary area of expertise is in artificial intelligence, with particular emphasis on human-AI interaction and collaboration and Explainable Artificial Intelligence (XAI). His work is at the intersection of artificial intelligence, interaction design, and cognitive science/psychology. He is an associate editor of the journal Artificial Intelligence.
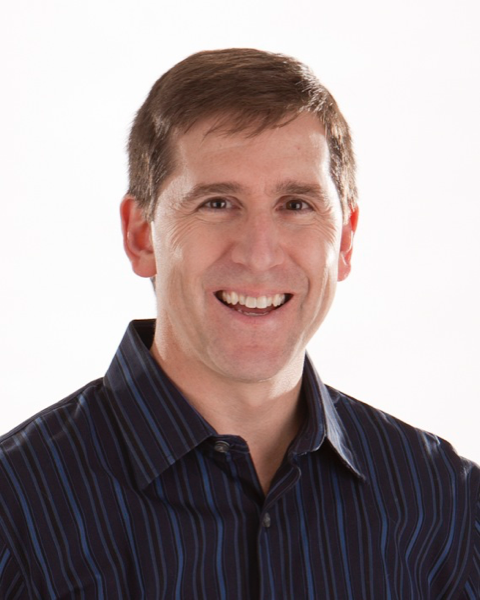
Pete Wurman
Title: Training the world’s best Gran Turismo racer
Abstract: Automobile racing represents an extreme example of real-time decision making in complex physical environments. Drivers must execute complex tactical maneuvers to pass or block opponents while operating their vehicles at their traction limits. Modern racing simulations, such as the PlayStation game Gran Turismo, faithfully reproduce much of the nonlinear control challenges of real race cars while also encapsulating the complex multi-agent interactions. In this talk I will describe how our team at Sony AI trained agents for Gran Turismo that can compete with the world’s best e-sports drivers. We combine state-of-the-art model-free deep reinforcement learning algorithms with mixed scenario training to learn an integrated control policy that combines exceptional speed with impressive tactics. In addition, we construct a reward function that enables the agent to be competitive while adhering to racing’s important, but under-specified, sportsmanship rules. We demonstrate the capabilities of our agent, Gran Turismo Sophy, by winning a head-to-head competition against four of the world’s best Gran Turismo drivers.
Bio: Pete Wurman is the director of Sony AI America and project lead for the Gran Turismo Sophy project. He has done research in computational auctions, multi-agent systems, robotics, and reinforcement learning. He earned his Ph.D. at the University of Michigan and was a professor at North Carolina State University. He was the Co-founder and CTO of Kiva Systems, the company that pioneered the use of mobile robotics in warehouse fulfillment operations. Kiva was acquired by Amazon in 2012 and, as Amazon Robotics, has deployed more than 500,000 robots to warehouses around the world. While at Amazon, Pete founded the Amazon Picking Challenge. He is an IEEE Fellow and a AAAI Fellow and in 2022 was inducted into the National Inventors Hall of Fame.
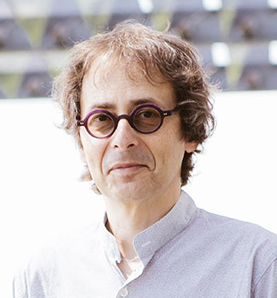
Jérôme Lang
CNRS, Université Paris-Dauphine PSL, France (sponsored by EurAI)
Title: From AI to social choice
Abstract: Computational social choice is an interdisciplinary field at the crossing point of economics, AI, and more generally computer science. It consists in designing, analysing and computing mechanisms for making collective decisions, with various application subfields such as voting, fair allocation of resources, participatory budgeting, selecting representative groups of individuals, or matching with preferences.
AI meeting social choice has not only lead to developing algorithms for collective decision making: it has helped reshaping and revitalising the field, by identifying new paradigms, new problems, new objects of study. In the talk I will give some examples showing the role of various AI sub-communities in the study of collective decision making.
Bio: Jérôme Lang is a CNRS senior scientist. He works at LAMSADE, Université Paris-Dauphine PSL. He has worked in several subfields of AI, especially knowledge representation and reasoning as well as multi-agent systems. His current main focus is computational social choice. He has published over 200 papers in leading AI conferences and journals. He was awarded a CNRS silver medal in 2017, and a Humboldt Research Award in 2020. He has served as a program chair of IJCAI-ECAI-2018, as well as COMSOC, KR, and TARK.
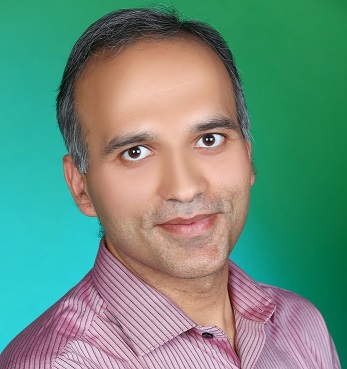
Sumit Gulwani
Title: AI-assisted Programming
Abstract: AI can enhance programming experiences for a diverse set of programmers: from professional developers and data scientists (proficient programmers) who need help in software engineering and data wrangling, all the way to spreadsheet users (low-code programmers) who need help in authoring formulas, and students (novice programmers) who seek hints when stuck with their programming homework. To communicate their need to AI, users can express their intent explicitly—as input-output examples or natural-language specification—or implicitly—where they encounter a bug (and expect AI to suggest a fix), or simply allow AI to observe their last few lines of code or edits (to have it suggest the next steps).
The task of synthesizing an intended program snippet from the user’s intent is both a search and a ranking problem. Search is required to discover candidate programs that correspond to the (often ambiguous) intent, and ranking is required to pick the best program from multiple plausible alternatives. This creates a fertile playground for combining symbolic-reasoning techniques, which model the semantics of programming operators, and machine-learning techniques, which can model human preferences in programming. Recent advances in large language models like Codex offer further promise to advance such neuro-symbolic techniques.
Finally, a few critical requirements in AI-assisted programming are usability, precision, and trust; and they create opportunities for innovative user experiences and interactivity paradigms. In this talk, I will explain these concepts using some existing successes, including the Flash Fill feature in Excel, Data Connectors in PowerQuery, and IntelliCode/CoPilot in Visual Studio. I will also describe several new opportunities in AI-assisted programming, which can drive the next set of foundational neuro-symbolic advances.
Bio: Sumit Gulwani is a computer scientist connecting ideas, people, and research & practice. He invented the popular Flash Fill feature in Excel, which has now also found its place in middle-school computing textbooks. He leads the PROSE research and engineering team at Microsoft that develops APIs for program synthesis and has incorporated them into various Microsoft products including Visual Studio, Office, Notebooks, PowerQuery, PowerApps, PowerAutomate, Powershell, and SQL. He is a sponsor of storytelling trainings and initiatives within Microsoft. He has started a novel research fellowship program in India, a remote apprenticeship model to scale up impact while nurturing globally diverse talent and growing research leaders. He has co-authored 11 award-winning papers (including 3 test-of-time awards from ICSE and POPL) amongst 140+ research publications across multiple computer science areas and delivered 60+ keynotes/invited talks. He was awarded the Max Planck-Humboldt medal in 2021 and the ACM SIGPLAN Robin Milner Young Researcher Award in 2014 for his pioneering contributions to program synthesis and intelligent tutoring systems. He obtained his PhD in Computer Science from UC-Berkeley, and was awarded the ACM SIGPLAN Outstanding Doctoral Dissertation Award. He obtained his BTech in Computer Science and Engineering from IIT Kanpur, and was awarded the President’s Gold Medal.
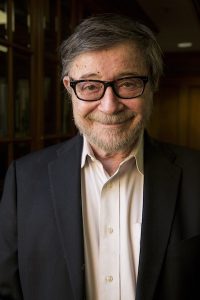
Judea Pearl
Title: What is Causal Inference and Where is Data Science Going?
Abstract: The availability of massive amounts of data coupled with impressive performance of machine learning algorithms has turned data-science into one of the most active research area in academia.
The past few years, however, have uncovered basic limitations in the model-free direction that data science has taken. An increasing number of researchers have come to realize that statistical methodologies and the “black-box” data-fitting strategies used in machine learning are too opaque and brittle, and must be enriched by a Causal Inference component to achieve their stated goal: Extract knowledge from data. Interest in Causal Inference has picked up momentum, and it is now one of the hottest topics in data science.
The purpose of this talk is to review what Causal Inference is all about, how it can be harnessed to solve practical data-scientific problems that cannot be solved by traditional methods, and why it promises to hold the key to the future of data science.
After summarizing some glaring deficiencies of “data fitting” methods, I will contrast them with “model-based” approaches and demonstrate how the latter can achieve a state of knowledge we can call “Deep Understanding”, that is, the capacity to answer questions of three types: predictions, interventions and counterfactuals.
I will further describe a computational model that facilitates reasoning at these three levels,
and demonstrate how features normally associated with “understanding” follow
from this model. These include generating explanations, generalizing across
domains, integrating data from several sources, assigning credit and blame, recovering from missing data, and more. I will conclude by describing future research directions, including automated scientific explorations and personalized decision-making.
Background Material:
https://ucla.in/3d2c2Fi
https://ucla.in/3iEDRVo
https://ucla.in/2HI2yyx
Bio: Judea Pearl is Chancellor professor of computer science and statistics and director of the Cognitive Systems Laboratory at UCLA, where he conducts research in artificial intelligence, human reasoning and the philosophy of science.
He is the author of Heuristics (1983) Probabilistic Reasoning (1988) and Causality (2000,2009) and a founding editor of the Journal of Causal Inference.
Among his awards are the Lakatos Award in the philosophy of science, The Allen Newell Award from the Association for Computing Machinery,
the Benjamin Franklin Medal, the Rumelhart Prize from the Cognitive Science Society, the ACM Turing Award, the Grenander Prize from the
American Mathematical Society, and the 2022 BBVA Frontier of Knowledge Award. He is the co-author (with Dana MacKenzie) of The Book of Why: The New Science of Cause and Effect which brings Causal Inference to a general audience.

Mihaela van der Schaar
Title: Panning for insights in medicine and beyond: New frontiers in machine learning interpretability
Abstract: Medicine has the potential to be transformed by machine learning (ML) by addressing core challenges such as time-series forecasts, clustering (phenotyping), and heterogeneous treatment effect estimation. However, to be embraced by clinicians and patients, ML approaches need to be interpretable. So far though, ML interpretability has been largely confined to explaining static predictions.
In this keynote, I describe an extensive new framework for ML interpretability. This framework allows us to 1) interpret ML methods for time-series forecasting, clustering (phenotyping), and heterogeneous treatment effect estimation using feature and example-based explanations, 2) provide personalized explanations of ML methods with reference to a set of examples freely selected by the user, and 3) unravel the underlying governing equations of medicine from data, enabling scientists to make new discoveries.
Bio: Mihaela van der Schaar is the John Humphrey Plummer Professor of Machine Learning, Artificial Intelligence and Medicine at the University of Cambridge and a Fellow at The Alan Turing Institute in London. In addition to leading the van der Schaar Lab, Mihaela is founder and director of the Cambridge Centre for AI in Medicine (CCAIM).
Mihaela was elected IEEE Fellow in 2009. She has received numerous awards, including the Oon Prize on Preventative Medicine from the University of Cambridge (2018), a National Science Foundation CAREER Award (2004), 3 IBM Faculty Awards, the IBM Exploratory Stream Analytics Innovation Award, the Philips Make a Difference Award and several best paper awards, including the IEEE Darlington Award.
Mihaela is personally credited as inventor on 35 USA patents (the majority of which are listed here), many of which are still frequently cited and adopted in standards. She has made over 45 contributions to international standards for which she received 3 ISO Awards. In 2019, a Nesta report determined that Mihaela was the most-cited female AI researcher in the U.K.
Ana Paiva
University of Lisbon, Portugal
Title: Engineering sociality and collaboration in AI systems
Abstract: Social agents, chatbots or social robots have the potential to change the way we interact with technology. As they become more affordable, they will have increased involvement in our daily activities with the ability to perform a wide range of tasks, and thus, partner with humans socially and collaboratively. But how do we engineer sociality and collaboration?
How can we build social agents that are able to “team up” with humans? How do we guarantee that these agents are trustworthy? To investigate these ideas we must seek inspiration in what it means to be a teammate and build the technology to support hybrid teams of humans and AI. That involves creating AI systems, agents and robots, which have capabilities such as social perception, interpersonal communication, collaboration and social responsibility.
In this talk, I will discuss how to engineer social and collaborative agents that act autonomously as members of a team, collaborating with both humans and other agents. Using case studies, I will discuss the challenges, recent results, and the future directions for the field of social and collaborative AI.
Bio: Ana Paiva is a Full Professor in the Department of Computer Engineering at Instituto Superior Técnico (IST) from the University of Lisbon and former Coordinator of GAIPS – “Group on AI for People and Society” at INESC-ID. Prof. Ana Paiva was also a 2020–2021 fellow at the Radcliffe Institute for Advanced Study at Harvard University. Prof. Paiva’s addresses problems and develops techniques for creating social agents that can simulate human-like behaviors and interact with humans in a natural and social manner. Over the years she has addressed this problem by engineering agents that can exhibit specific social communication competencies, including affective communication, culture, non-verbal behavior, empathy, collaboration, and others. Her more recent research combines methods from AI, social robotics and social modelling to study hybrid societies of humans and machines, in particular to engineer agents that lead to more cooperative, prosocial and altruistic societies. She has published extensively and received best paper awards in different conferences, in particular she won the first prize of the Blue Sky Awards at the AAAI in 2018. She has further advanced the area of artificial intelligence and social agents worldwide, having served for the Global Agenda Council in Artificial Intelligence and Robotics of the World Economic Forum and as a member of the Scientific Advisory Board of Science Europe. She is an EurAI fellow, an ELLIS fellow and member of the extended core group of the CLAIRE (Confederation of Laboratories for AI research in Europe).